With Phi-2, Microsoft is getting down to SMLS
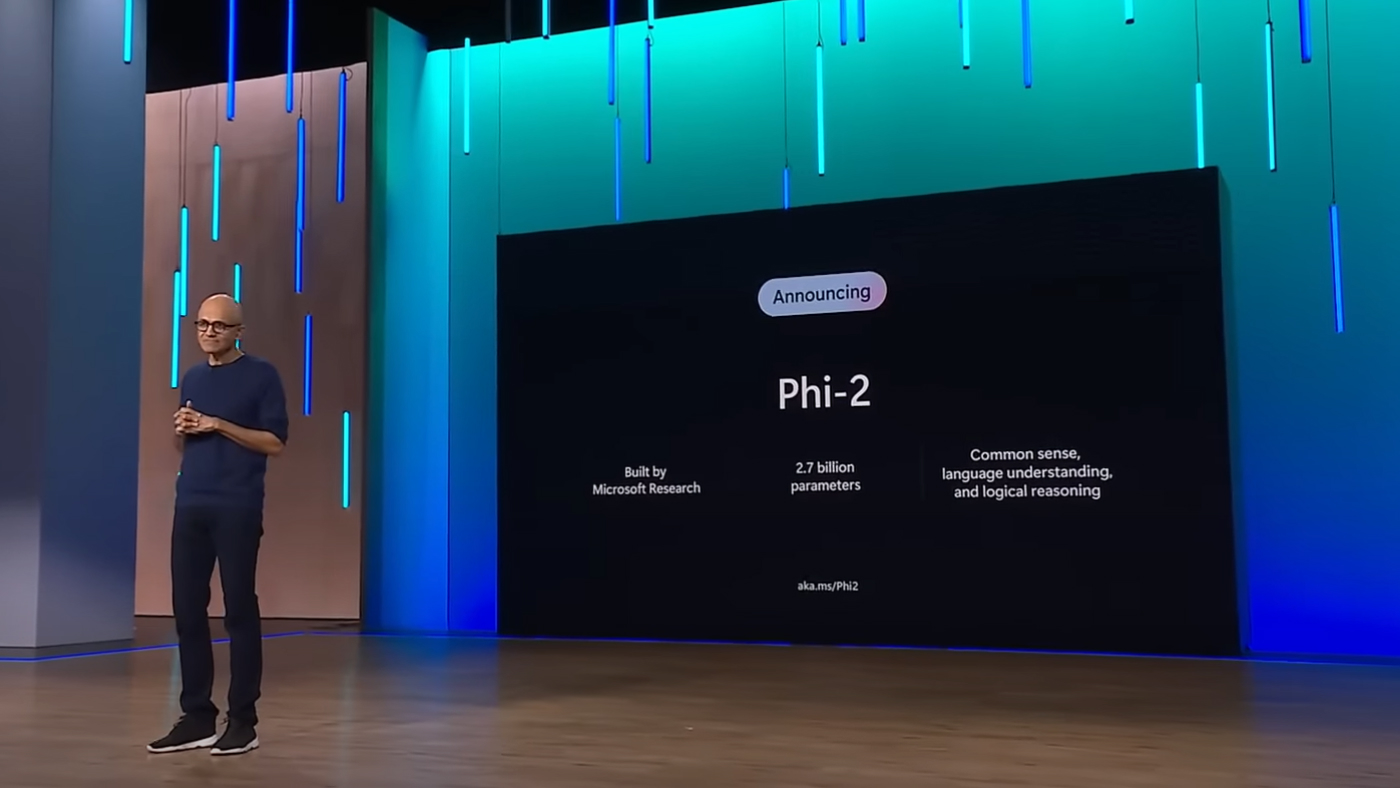
Picture: Microsoft.
When we talk about language models and generative AI (AI), we usually think of large language models, also called LLM (for Large Language Model). These LLMs power the most popular chatbots, ChatGPT, Bard and Copilot, not to name them. However, the new language model revealed by Microsoft is proof that small language models (SLMs) also have a future in the field.
This Wednesday, Microsoft launched Phi-2, a small language model capable of reasoning and understanding our language. The template is now available in the Azure AI Studio Template catalog.
A big one among the little ones
From “small” he has only the name. Phi-2 contains 2.7 billion parameters in its model, which represents a big leap forward compared to Phi-1.5, which had “only” 1.3 billion.
Although it is compact, the new Microsoft model displays “peak performance” among these congeners of less than 13 billion parameters. It even outperforms models up to 25 times larger in complex reference tests, according to its manufacturer.
Phi-2 surpasses in particular the Llama-2 models from Meta, Mistral, and even Gemini Nano 2, the smallest version of Google’s most powerful LLM, Gemini on several reference points :
Picture: Microsoft.
Train SLMs comparable to larger models
The performance of the new language model is in line with the objectives that Microsoft has set with Phi, namely to develop an SLM with emerging capabilities and performance comparable to that of larger-scale models.
“It remains to be seen whether such emerging capabilities can be obtained on a smaller scale by using strategic choices for training – for example, data selection,” Microsoft nuance. “Our work on Phi models aims to answer this question by forming SLMs that achieve performance comparable to that of larger-scale models (although they are still far from the most widespread models). »
During the training of Phi-2, Microsoft was very selective about the data used. The company first used what it calls “handwritten quality” data. Microsoft then enriched its database by adding carefully selected web data, filtered according to their educational value and the quality of their content.
Why this interest in SLM?
SLMs are a cost-effective alternative to LLMs. These smaller models are also useful for performing less demanding tasks, which do not need the power of an LLM.
In addition, the computing power required to operate SLMs is much lower than that of LLMs. This reduced requirement means that users do not necessarily need to invest in expensive GPUs to meet their data processing needs.
Source: ZDNet.com