Integrating ARKit with Machine Learning: Creating Intelligent Augmented Reality Apps
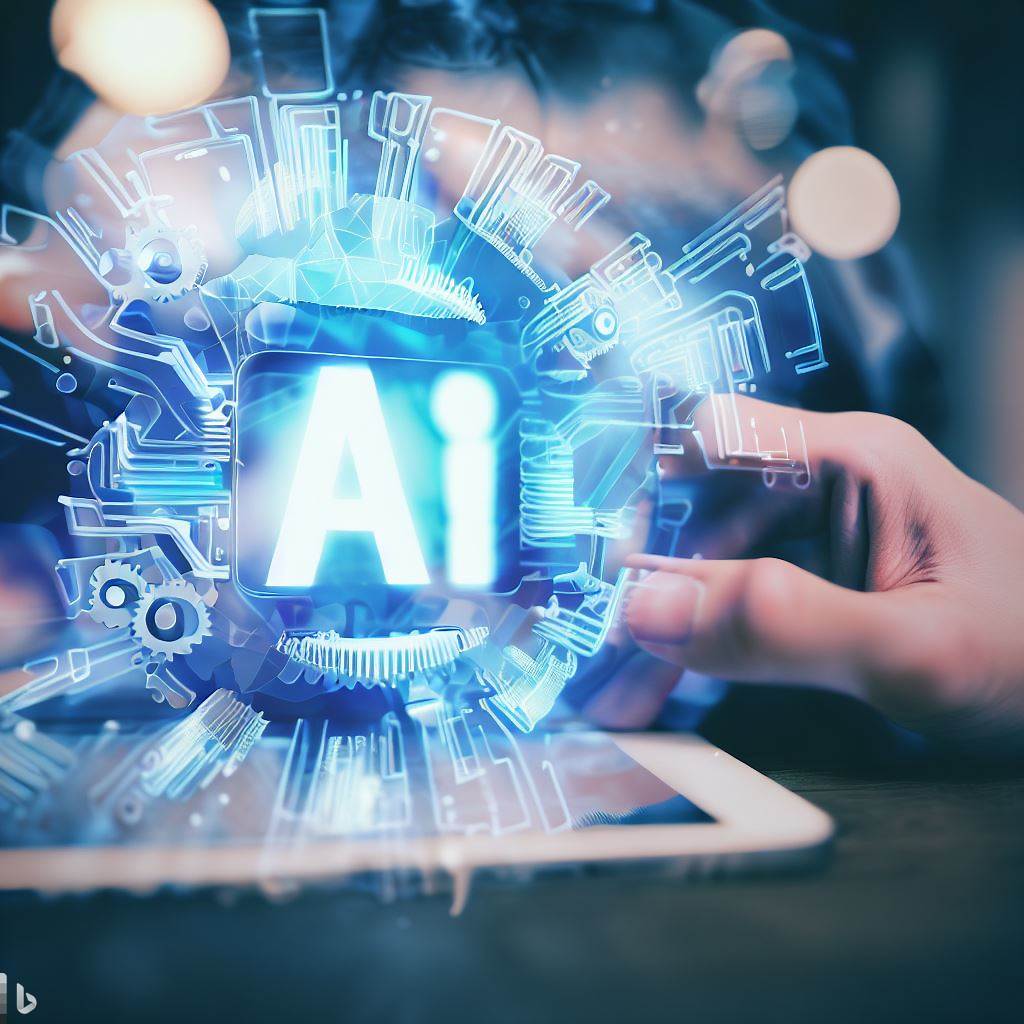
Augmented Reality (AR) has transformed the way we interact with digital content, and combining it with Machine Learning (ML) opens up a whole new world of possibilities. Apple’s ARKit, coupled with the power of machine learning algorithms, enables the creation of intelligent AR apps that can recognize, understand, and interact with the real world in a more sophisticated and personalized manner. In this article, we will explore the potential of integrating ARKit with machine learning and showcase how this fusion can lead to the development of intelligent augmented reality apps.
- Object Recognition and Tracking: Machine learning algorithms can be used in conjunction with ARKit to enable object recognition and tracking. By training ML models with annotated data, AR apps can identify and track specific objects or images in the real world. This integration allows virtual objects to interact seamlessly with recognized objects, creating immersive and contextually relevant AR experiences. For example, an AR app could recognize a particular product or artwork and overlay additional information or interactive elements onto it.
- Gesture and Pose Recognition: Integrating machine learning with ARKit can enhance gesture and pose recognition capabilities. ML models can be trained to identify and interpret specific hand movements or body poses, enabling users to interact with AR content through natural gestures. This fusion allows for more intuitive and immersive AR experiences, where users can control virtual objects or navigate through the AR environment using gestures or body movements.
- Scene Understanding and Spatial Mapping: Machine learning algorithms can assist in scene understanding and spatial mapping, providing ARKit with the ability to recognize and understand the 3D structure of the environment. By analyzing visual data from the device’s camera, ML models can infer the layout of the scene, detect surfaces, and understand the spatial relationships between objects. This integration enables virtual objects to interact realistically with the physical environment, creating a more immersive and cohesive AR experience.
- Personalized Recommendations and Contextual Information: By leveraging machine learning algorithms, ARKit can deliver personalized recommendations and contextual information to users based on their preferences, behaviors, and real-time environmental data. ML models can analyze user data and make predictions or suggestions tailored to individual users, providing them with relevant product recommendations, location-based information, or personalized content within the AR app. This integration enhances user engagement and delivers a more personalized and interactive AR experience.
- Real-Time Object Detection and Tracking: Machine learning algorithms can be used to enable real-time object detection and tracking within ARKit apps. This integration allows AR apps to identify and track objects dynamically, enabling virtual objects to interact with the recognized objects in real-time. For instance, an AR app can detect and track the movements of a user’s face, allowing virtual masks or filters to accurately align and respond to the user’s expressions in real-time.
Conclusion: Integrating ARKit with machine learning unlocks a world of possibilities for creating intelligent augmented reality apps. By leveraging ML algorithms for object recognition and tracking, gesture and pose recognition, scene understanding and spatial mapping, personalized recommendations, and real-time object detection, developers can create more immersive, interactive, and contextually relevant AR experiences. This fusion of ARKit and machine learning enables intelligent AR apps that can understand and interact with the real world in a more sophisticated and personalized manner. Embrace the potential of integrating ARKit with machine learning to create innovative and intelligent augmented reality apps that provide users with immersive and tailored experiences.