Leveraging real-time data to unify generative AI and predictive AI
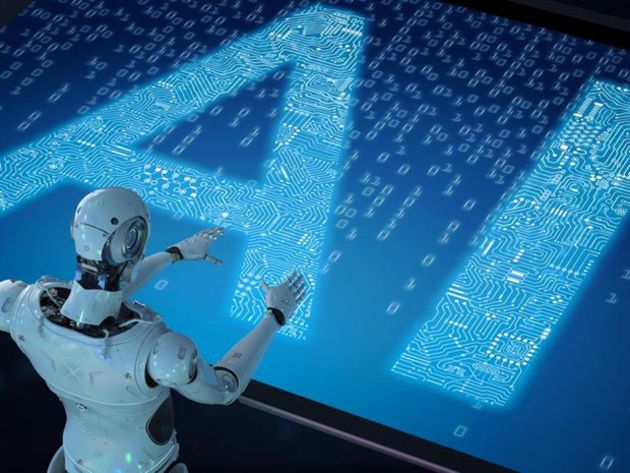
In the age of data-driven (data-based decision-making), the role of artificial intelligence (AI) is fundamental. Whether it’s predicting stock market trends or generating personalized content for users, AI models are essential. However, the effectiveness of these models is closely linked to the quality and relevance of the data they exploit.
Outdated data and its impact on predictive results
The adage “garbage in, garbage out” (what comes in comes out) is confirmed in the field of AI. When models are designed or powered from incomplete, biased or outdated information, predictive results are impacted. For example, in financial markets where conditions change in a few milliseconds, relying on outdated data can cause opportunities to be missed, or even cause sometimes colossal financial losses. Outdated data gives the illusion of accuracy. The models can then show great confidence in their predictions, but these are based on a reality that no longer exists.
Overview of the possible consequences related to the use of outdated data :
- Commercial decisions: In finance, healthcare or even retail, decisions based on outdated data can lead to errors in orders or restocking, unwise investments and investments, and in fine, significant financial losses.
- Safety concerns: In certain critical areas related to everyone’s safety, such as autonomous driving or medical diagnostics, outdated data can be a matter of life or death.
- Consumer experience: As part of a customer-centric service offering, such as online recommendation systems or personalized marketing, predictions based on outdated data would produce a decrease in user engagement and satisfaction, or even repercussions on the company’s reputation, especially through its image on social networks.
Overcoming the reality of hallucinations in fundamental models
Large artificial intelligence models are incredibly powerful, but they are also likely to generate meaningless or incorrect content, a phenomenon known as “hallucination” in fundamental models. These hallucinations occur because the model relies on a standard dataset that does not necessarily contain the most recent or contextually relevant information.
The integration of real-time data into the algorithm of large models can drastically reduce the frequency of hallucinations. When the model has access to the most recent data, it can generate predictions or contextually relevant content. This is an essential element for companies that want to take advantage of the power of AI to promote real-time decision-making, and access the predictive power with high added value that AI can offer.
The role of databases in real-time AI
The foundation for creating hypercontextualized and personalized experiences for generative applications enriched by AI is found in the organization’s document and control system. Real-time data is an integral part of this real-time AI application stack, and it is imperative that operational databases are tightly integrated into the AI pipeline.
In order to create these experiences, it is necessary to be able to guarantee developers access to an efficient multi-model database platform, capable of storing large volumes, and allowing unstructured data to be managed and searched efficiently. They need a long-term memory layer for large language models (LLM), in order to offer answers that take into account the context of real—time data, as well as conversational history, and have the ability to store and search data in the native LLM format – that of unstructured data, managed by high-dimensional vector databases.
The future of generative and predictive AI
Real-time data, combined with unstructured storage and search capacity, can give fundamental models their long-term memory. Such databases can contain large amounts of information and make them easily accessible to the model, thus acting as the “memory” of the model.
The integration of real-time data into generative and predictive AI models is not only a technical improvement, but also a change in philosophy. As we move towards a world where changes are ever more frequent, the ability of AI to adapt and provide accurate and contextualized information will be the sine qua non for effective decision-making. By overcoming the challenges posed by outdated data and hallucinations, the true potential of AI can be unleashed, which will make it an invaluable asset for data-driven strategies of the future.