SeamlessM4T: How to use Meta’s new AI-powered multilingual translator
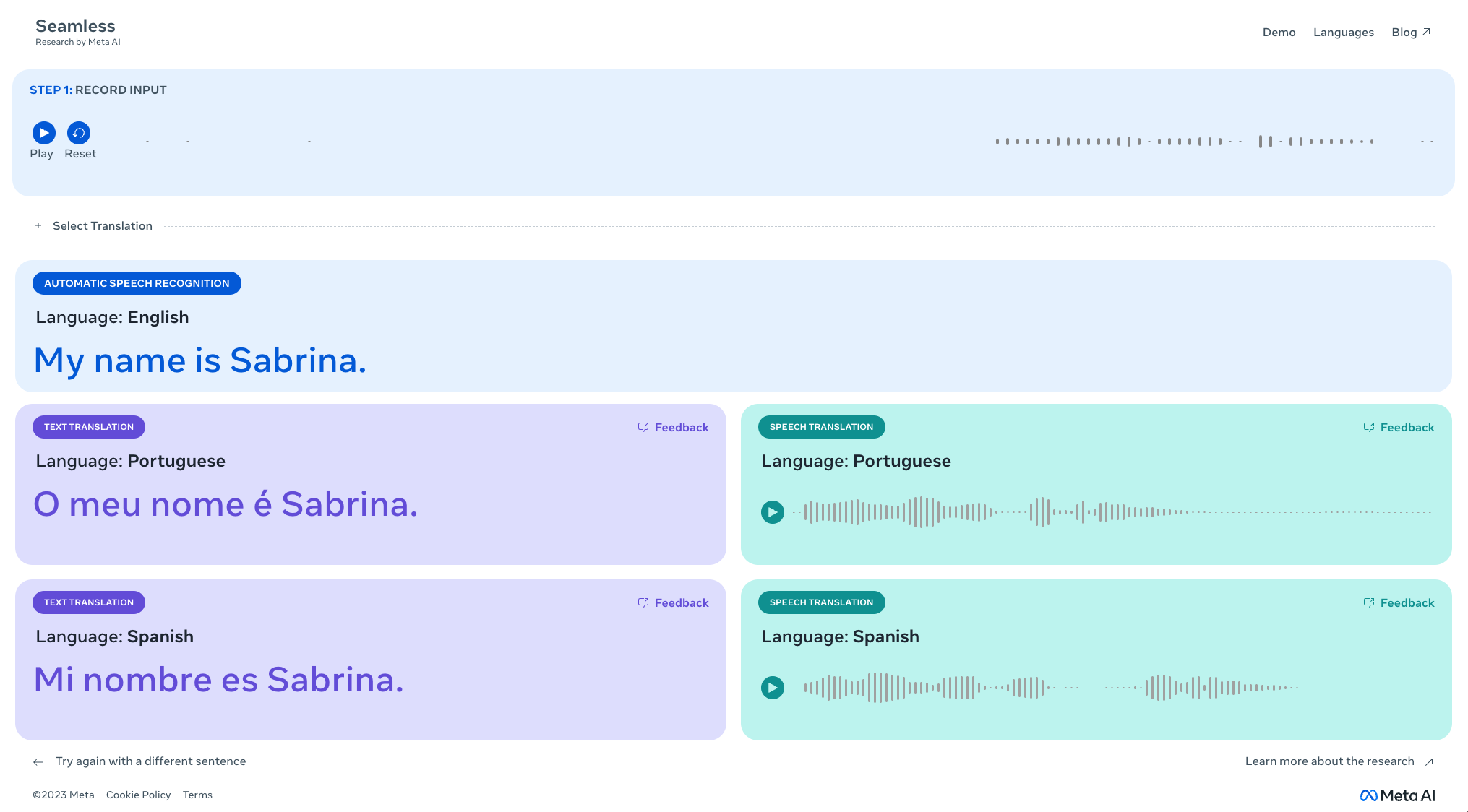
A few seconds after recording my audio, the model produced the text translation accompanied by an audio translation. Screenshot by Sabrina Ortiz/ZDNET
Adios Google Translate. Bis später. Hasta luego.
Learning another language is difficult. Meta’s new AI translation model is here to do the heavy lifting for you, and you can even try a demo.
On Tuesday, Meta announced SeamlessM4T, the first all-in-one multimodal and multilingual translation AI model that supports almost 100 languages. The model can perform speech-to-text, speech-to-speech, text-to-speech and text-to-text translations. Meta claims that SeamlessM4T’s single-system approach reduces errors and delays, thereby increasing translation efficiency and quality.
Select up to three languages in which you want the sentence to be translated
To try the model, all you have to do is open this demo link in your browser and save a complete sentence that you want to have translated. For best results, Meta recommends trying in a quiet environment.
Then you can select up to three languages in which you want the sentence to be translated. Once you have entered your sentence, you can view a transcript and listen to the translations.
I tried the demo and was impressed by the accuracy and speed of the results. A few seconds after recording my sentence, the model produced a text translation accompanied by an audio translation, as shown in the photo above.
Countdown to the great linguistic models
As this is a demonstration, Meta warns that it may produce inaccurate translations or change the meaning of the words entered. If users encounter these inaccuracies, Meta encourages them to use the feedback function to report errors so that the model can be improved.
Meta claims that SeamlessM4T surpasses existing translation models that are trained specifically for speech-to-speech translation between languages, as well as models that convert speech and text into several language pairs. SeamlessM4T is an example of what is called multimodality, that is, the ability of a program to operate on several types of data, in this case voice and text data.
Previously, Meta had focused on large linguistic models capable of translating text between 200 different languages. This focus on the text poses a problem, according to Loïc Barrault, from Meta.
Tackling the translation of speech
“While unimodal models such as No Language Left Behind (NLLB) bring the coverage of text-to-text translation (T2TT) to more than 200 languages, unified S2ST (speech-to-speech-to-text) models are far from achieving similar scope or performance,” writes.
The official document, “SeamlessM4T — Massively Multilingual & Multimodal Machine Translation”, is published on the website dedicated to the Meta project, Seamless Communication. There is also a related GitHub site.
Speech was left out at the time in part because there was less voice data available in the public domain to train neural networks, the authors write. But above all because voice data is richer to process as a signal for neural networks.
“Speech is more difficult to process from the point of view of machine translation”
“Speech is more difficult to process from the point of view of machine translation. It encodes more information. This is also the reason why she is superior at conveying intention and forging stronger social ties between the interlocutors,” Barrault writes.
The objective of SeamlessM4T is to create a program that is trained on both voice data and text data.
And it should be noted that multimodality is an explicit element of the program.
Such a program is sometimes called an “end-to-end” program because it does not divide the parts concerning the text and the parts concerning the speech into separate functions, as in the case of “cascade models”, where the program is first trained on an element, such as the conversion of speech into text, then on another element, such as the conversion of speech into speech.
As the authors of the program say, “most S2ST systems today are based on cascade systems consisting of several subsystems that perform translation gradually – for example, from automatic speech recognition (ASR) to T2TT, and then to text-to-speech synthesis (TTS) in a three-step system”.
A program that combines several existing parts trained together
Instead, the authors built a program that combines several existing parts trained together. They have included “SeamlessM4T-NLLB, a massively multilingual T2TT model”, as well as a program called w2v-BERT 2.0, “a speech representation learning model that exploits unlabeled voice audio data”, plus T2U, “a text-to-unit sequence model”, and HiFi-GAN multilingual, a “unit vocoder to synthesize speech from units”.
The four components are assembled like a Lego set in a single program, also introduced this year by Meta, called UnitY, which can be described as “a two-step modeling framework that first generates text and then predicts discrete acoustic units”.
The entire organization is visible in the diagram below.
The authors have built a program that combines several existing parts formed together, which are all plugged together like a set of Lego in a single program. Meta AI Search 2023
The program manages to do better than many other types of programs when testing speech recognition, voice translation and speech-to-text conversion, the authors report. In particular, he beat alteration programs that are also end-to-end, as well as programs designed explicitly for speech recognition.
The accompanying GitHub site offers not only the program code, but also SONAR, a new technology for “integrating” multimodal data, and BLASAR 2.0, a new version of a metric allowing multimodal tasks to be evaluated automatically.
Sources: “ZDNet.com ” and “ZDNet.com “