What is generative AI and why is it so popular? Here’s everything you need to know
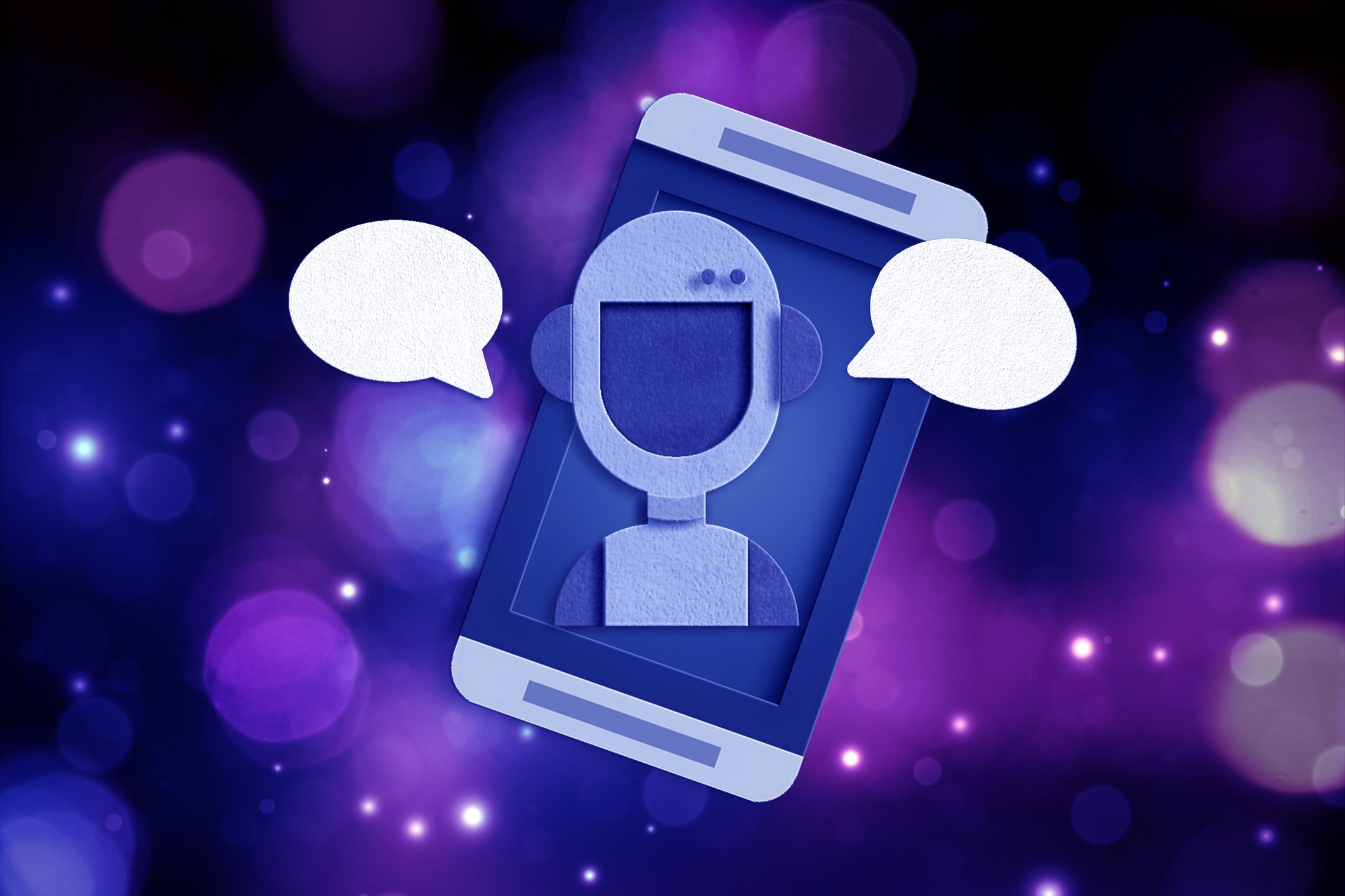
Generative AI is obviously a hot topic. But what is it concretely? Here we have some answers.
What is generative AI?
Generative AI refers to models or algorithms that create new results, such as text, photos, videos, code, data or 3D renderings, from the large amounts of data on which they have been trained.
The models “generate” new content by referring to the data on which they have been trained and making new predictions.
The objective of generative AI is to create content, unlike other forms of AI, which can be used for different purposes, such as data analysis or assistance in driving an autonomous car.
Why is generative AI a hot topic?
The term “generative AI” is being talked about due to the growing popularity of generative AI programs, such as OpenAI’s ChatGPT conversational chatbot and the DALL-E image generator. These and other similar tools use generative AI to create added value. These and other similar tools use generative AI to produce new content, including computer code, essays, emails, social media captions, images, poems, Excel formulas and much more in a matter of seconds. Enough to upset the way people do things currently.
ChatGPT has become extremely popular, accumulating more than a million users a week after its launch. Many other companies have also entered the competition, including Google, Microsoft’s Bing and Anthropic. The craze around generative AI will certainly continue to grow as other companies join them and find new use cases as the technology becomes more integrated into everyday processes.
What is the relationship between machine learning and generative AI?
Machine learning refers to a dimension of AI that teaches a system to make a prediction based on data on which it has been trained. An example of this type of prediction is when DALL-E is able to create an image from the prompt that you enter by discerning what the prompt actually means.
So generative AI is a machine learning framework, but not all machine learning frameworks are made for generative AI.
What are the systems that use generative AI?
Generative AI is used in any AI algorithm or model that uses AI to produce a new attribute. The most prominent examples are ChatGPT and DALL-E.
However, after seeing the buzz around generative AI, many companies have developed their own generative AI models. This list of tools, which is constantly growing, includes, among others, Google Bard, Bing Chat, Claude, PaLM 2, LLaMA, etc.
What is art in generative AI?
Creating art with generative AI consists of creating works with AI models trained on existing works of art. The model is trained on billions of images found on the Internet. The model uses this data to learn the styles of images and then uses this knowledge to generate new works of art when a person asks him through a text.
A popular example of an AI art generator is DALL-E. However, there are many other AI generators on the market that are just as good, or even more efficient, and that can be used for different needs. Bing Image Generator is Microsoft’s version of this technology, which exploits a more advanced version of DALL-E 2, for example.
What are text-based generative AI models trained on?
Text-based models, such as ChatGPT, are trained on massive amounts of text as part of a process known as self-supervised learning. The model learns from the information provided to it to make predictions and provide answers in the future.
Generative AI models, especially those that generate text, pose a problem because they are formed from data from all over the internet. This data includes copyrighted material and information that may not have been shared with the consent of their owner.
What are the implications of generative AI in the field of art?
The artistic models of generative AI are trained on billions of images from all over the internet. These images are often works of art produced by a specific artist, which are then reimagined and reassigned by the AI to generate your image.
Although it is not the same image, the new image has elements of the artist’s original work, which are not attributed to him. A specific style specific to the artist can therefore be reproduced by the AI and used to generate a new image, without the original artist knowing or approving it. The debate about whether AI-generated art is actually “new” or even “artistic” will probably continue for many years.
What are the shortcomings of generative AI?
Generative AI models use a large amount of content from all over the internet, then use the information they have been trained on to make predictions and create a result for the prompt you enter. These predictions are based on the data provided to the models, but there is no guarantee that the prediction is correct, even if the answers seem plausible.
The answers can also incorporate biases inherent in the content that the model has ingested on the internet, but there is often no way to know if this is the case. These two shortcomings have raised serious concerns about the role of generative AI in spreading false information.
Generative AI models do not necessarily know if the information they produce is accurate and, most of the time, we have little way of knowing where the information comes from and how it has been processed by the algorithms to generate content.
There are many examples of chatbots, for example, that provide incorrect information or that simply invent things to fill in the gaps. While the results of generative AI can be intriguing and entertaining, it would be unwise, at least in the short term, to rely on the information or content they create.
Some generative AI models, such as Bing Chat or GPT-4, try to fill this lack of sources by providing footnotes with sources that allow users not only to know where their answer came from, but also to verify its accuracy.
Source: “ZDNet.com “